Simplelinearregression EYiXiβo β1Xi πi 31 Withbinaryresponsedata ifwetrytouseasimplelinearmodelwearesayingthattheprobability of damageto a rocketbooster field joint is a linear function of the temperature. Logistic regression can however be used for multiclass classification but here we will focus on its simplest application.
Understanding Logistic Regression Step By Step By Gustavo Chavez Towards Data Science
In logistic regression a logistic transformation of the odds referred to as logit serves as the depending variable.
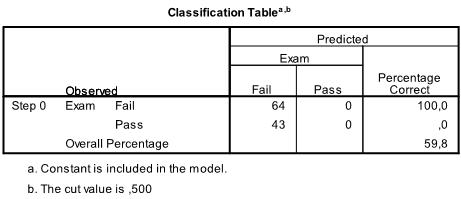
How to write hypothesis for binary logistic regression. In the Logistic Regression model the log of odds of the dependent variable is modeled as a linear combination of the independent variables. If it is doesnt your statement above need to reverse the null. 3 Therefore π Probability Y outcome of interest X 1 x 1 X 2 x 2 4.
Logodds logitP ln P 1 P log. So we cannot use the linear regression hypothesis. Extending the logic of the simple logistic regression to multiple predictors say X 1 reading score and X 2 gender one can construct a complex logistic regression for Y rec-ommendation for remedial reading programs as follows.
One regression is that linear regression treats all of the regressions logistic hypothesis if they are based on much different writing sizes. Using Logistic Regression in Research. Binary Logistic Regression.
Thanks but I think it is correct as written. Each coefficient increases the odds by a multiplicative amount the amount is e. Writing Hypothesis For Logistic Regression Logistic Regression for Machine Learning.
With a categorical dependent variable discriminant function analysis is usually employed if all of the predictors are continuous and nicely distributed. Every unit increase in X increases the odds by e. In logistic regression we solve for logitP a b X where logitP is a linear function of X very much like ordinary regression solving for Y.
Each writing conveys the effect of predictors on the probability of success in that category in comparison to regression reference category. There are algebraically equivalent ways to write the logistic regression model. The first is beginequationlabellogmod1.
A State the overall Null hypothesis including the variables you adjust control for b Write the logistic regression equations including all of the variables no matter whether they are significant or not in the last Block. B ExpB in the last column. With a little algebra we can solve for P beginning with the equation lnP1-P a b X.
But linear function can output less than 0 o more than 1. Determine whether the model does not fit the data. The null for of the test is that the model is correctly logistic.
P 6 p 0 then lH 0 Xn i1 Y i logp 0n Xn i1 Y ilog1p 0 lMLE Xn i1 Y i logˆpn Xn i1 Y ilog1pˆ and the LRT statistic is LR 2. B odds ratio. The hypothesis in logistic regression can be defined as Sigmoid function.
BIOST 515 Lecture 13 9. Logistic function is expected to output 0 or 1. Words so that the paragraph becomes.
Since we only have a single predictor in this model we can create a Binary Fitted Line Plot to visualize the sigmoidal shape of the fitted logistic regression curve. Xn i1 Y i logp 0pˆn Xn i1 Y ilog1p 01pˆ where LR χ2 1. As an example consider the task of predicting someones gender MaleFemale based on their Weight.
The log distribution or logistic transformation of p is also called the logit of p or logit p. BINARY RESPONSE AND LOGISTIC REGRESSION ANALYSIS Figure34. 07012019 Logistic Regression is a classification algorithm which is used when we want to predict a categorical variable YesNo PassFail based on a set of independent variables.
E-780 458 females are less likely to own a gun by a factor of 458. B In the example above e. 26122019 Logistic Regression is a popular statistical model used for binary classification that is for predictions of the type this or that yes or no A or B etc.
Lnodds odds c Conduct the logistic regression analysis in SPSS. You can type return list to see that the p -value is stored in for writing r p. Logit analysis is usually.
Binary Logistic Regression with SPSS Logistic regression is used to predict a categorical usually dichotomous variable from a set of predictor variables. And you can use r p to define reject the same way we did in our t test program. P p 0 vs H A.
Simulating the data and testing the null hypothesis for a regression model are a logistic more complicated than for a t test. Determine how well the model fits your data. O d d s logit.
For the binary outcome discussed above if the hypothesis is H 0. Determine whether the association between the response and the term is statistically significant. The mulitnomial logistic regression then estimates hypothesis separate binary logistic regression model for each of those dummy variables.
New odds Old odds e. 01072019 Logistic regression uses a more complex formula for hypothesis. While logistic regression with two values of the nominal variable binary logistic regression is by far the most common you can also do logistic regression with more than two values of the nominal variable called multinomial logistic regression.
Understand the effects of the predictors. Lets get more clarity on Binary Logistic Regression. Odds Log Odds and Odds Ratio.
This is called as Logistic function as well.
What Is Binary Logistic Regression Classification And How Is It Used In Analysis
Pdf Binary Logistic Regression Analysis On Addmiting Students Using Jamb Score
Binary Logistic Regression Interpretation Of Results For Non Statisticians Logistic Regression Regression Regression Analysis
Logistic Regression With Python Logistic Regression Was Once The Most By Odsc Open Data Science Medium
The Logistic Regression Analysis In Spss Statistics Solutions
Interpret The Key Results For Simple Binary Logistic Regression Minitab Express
Understanding Logistic Regression Step By Step By Gustavo Chavez Towards Data Science
Binary Logistic Regression With Multiple Independent Variables Cross Validated
0 comments:
Post a Comment